Applied Materials’ semi-conductor wafer processing tools and chambers generate significant amounts of time series data that is actively monitored for performance excursions using guard banding during chamber operation. However, there is a need for more advanced methods of time series data analysis that would include functions such as:
1) Data conditioning (start time alignment, noise filtering, moving average analysis)
2) Anomaly detection and classification
3) Pattern recognition/Feature identification (i.e. calculate slopes, find max, min, & inflections points, control system filtering, or FFT)
4) Fast comparison of multiple data streams
5) Compilation of items 1-4 above for future comparison and optimization via ML techniques. Preferably, item 5 (i.e. compilation) will utilize highly efficient storage methods for data retention with metrics to evaluate the efficiency of the storage requirement.
As there are many techniques for items 1-5, as a 6th key deliverable of the project we propose to compare 3 or more techniques to determine the best of breed with respect to analytical speed and computational resources consumed.
Applied Materials will provide a complete, anonymized set of time series data for analysis from an Applied Materials semi-conductor wafer processing tool and subject matter experts to navigate the data and evaluate the analysis results.
Deliverables of the project would include items 1-6 as outlined above presented by means of a user-friendly UI for data visualization.
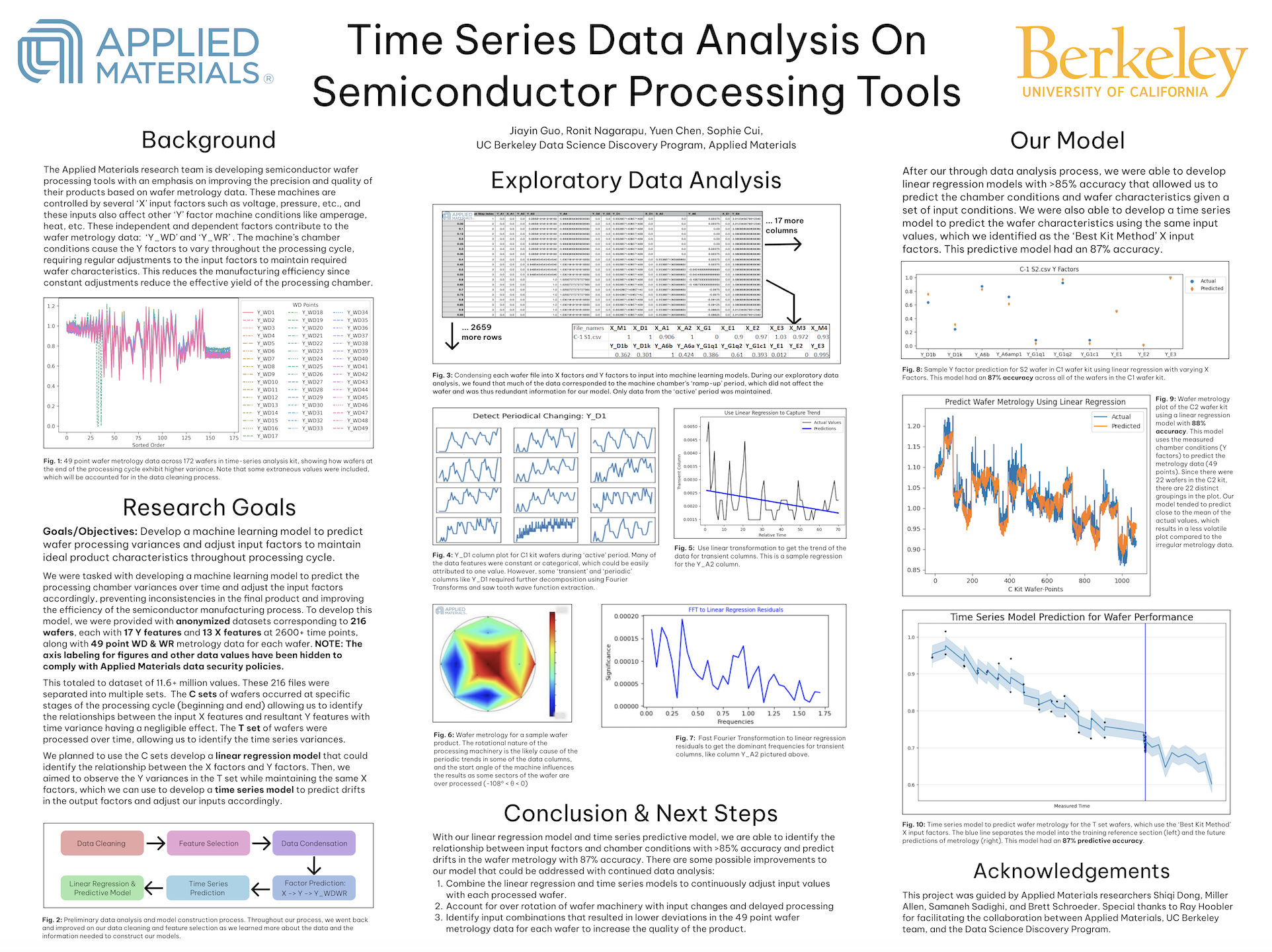